Detailseite für das News-Plug-In auf der Startseite
Predictive parametrization of thermodynamic models using Machine Learning
- Research Highlights
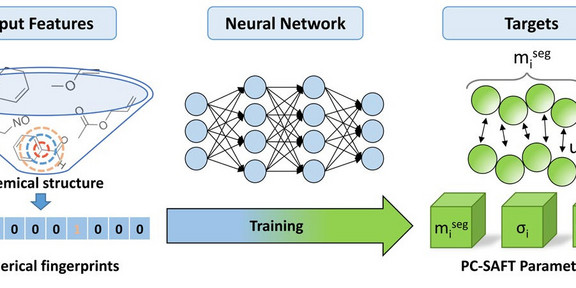
Accessing PC-SAFT pure-component parameters with neural network ensembles
Simulation of chemical or biotechnological processes is a key concept to efficiently design and optimize industrial processes or production plants. Thereby, thermodynamic phase equilibria of complex systems have to be available using advanced models, such as PC-SAFT. PC-SAFT requires the parametrization of each component, which is usually performed by fitting to experimental data. Acquiring experimental data is a time-consuming and costly procedure, for some components even unfeasible. In this work we used neural networks (NNs) to predict PC-SAFT pure-component parameters without the need of experimental data.
- ScientificHighlights_2022_JH.pdf PDF (183 KB)